Introduction
Audience analytics trends refers to the collection and analysis of data about audience behaviors, preferences, and engagement across various channels. In 2025, virtually every industry – from media and entertainment to retail and e-commerce – is leveraging audience analytics to drive decision-making and personalize experiences. The global audience analytics market itself is growing robustly, reflecting this rising demand for data-driven insights. In 2025, the market is estimated to be around $6–6.6 billion, up from about $5.4 billion in 2023.
Forecasts indicate it will nearly double by the end of the decade – reaching over $10 billion globally by 2029 – as companies continue investing in analytics capabilities.
Key drivers include the surge in digital content consumption, the need for personalized customer experiences, and advancements in AI and big data processing.
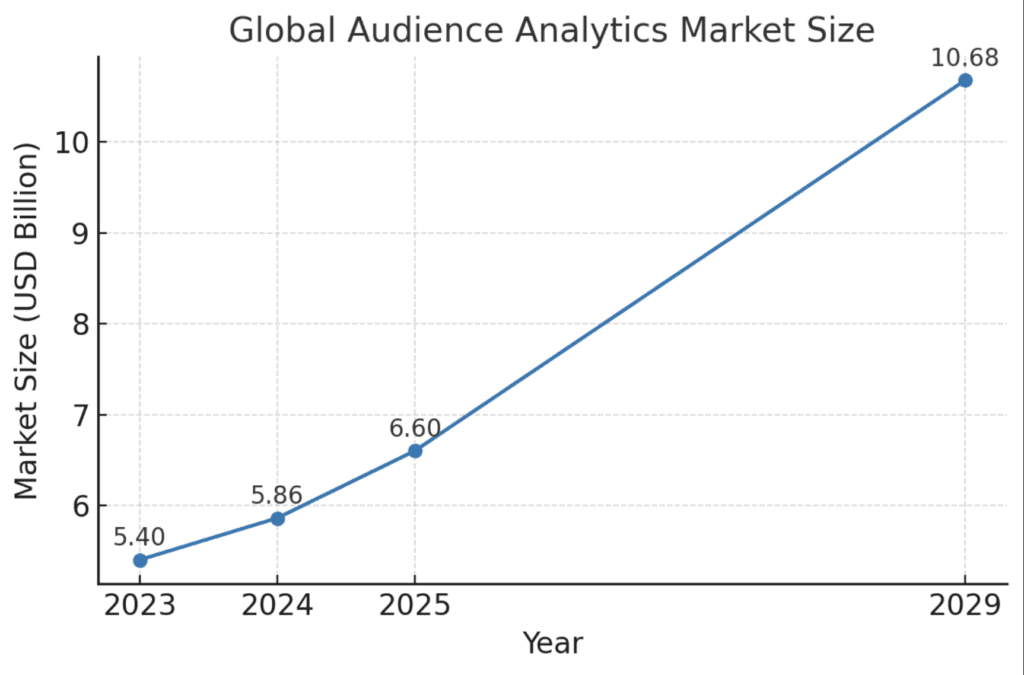
In this report, we provide a comprehensive overview of audience analytics trends in 2025, covering major sectors such as media and entertainment, digital content and social media, retail and e-commerce, events, and mobile apps (among others).
We will examine how each sector is utilizing analytics to understand and engage audiences, backed by real facts, figures, and industry forecasts. We also highlight case studies of leading companies and platforms that exemplify these trends, and include a competitive analysis of key players in the audience analytics landscape. Throughout, the focus is on clarity, depth, and factual accuracy – aligning with a user-centric, analytical narrative.
Market Overview and Key Trends in 2025
Audience analytics has become a strategic imperative for organizations in 2025. Several overarching trends are shaping this field across all industries:
Explosion of Digital Audiences
The world is more connected than ever, providing a massive data pool for analytics. Over 5.5 billion people (67.9% of the global population) were using the internet at the start of 2025, and social media use has likewise soared to 5.24 billion users (63.9% of the population).
Digital adoption growth
This has moderated in recent years (internet users grew +2.5% YoY as of Jan 2025), but the scale is unprecedented. Companies can now reach and analyze audiences at global scale, but this also means audience data is voluminous and highly diverse, coming from web, mobile, social platforms and more.
AI and Machine Learning Integration
AI is transforming how audience data is processed and utilized. In 2025, integrating AI/ML into analytics is a top trend, enabling advanced capabilities like predictive modeling, automated segmentation, and personalization at scale. For example, machine learning algorithms can sift through big datasets to find patterns in customer behavior that humans might miss.
By 2025, over 50% of retailers are expected to adopt AI-driven analytics solutions to predict consumer demand, personalize marketing, and optimize supply chains– and similar adoption rates are seen in media, finance, and other sectors. These AI-powered insights allow real-time responsiveness; companies can adjust content or offers immediately based on model predictions.
A narrative case is Netflix’s AI-driven recommendation engine, which analyzes viewing behavior to suggest content – this system is so effective at keeping users engaged that it saves Netflix an estimated $1 billion per year by reducing churn.
Real-Time and Streaming Analytics
Businesses increasingly seek real-time customer insights rather than retrospective reports. In fast-paced arenas like social media or e-commerce flash sales, the ability to analyze audience reactions instantaneously is critical. Modern analytics platforms are leveraging streaming data and in-memory computing to provide live dashboards of audience activity.
For instance, streaming video platforms track viewer drop-off second by second; retailers monitor website clickstreams in real time to detect funnel fallouts. This real-time focus is evident in the market – by 2026, an estimated 35% of retailers will use real-time analytics for inventory and supply chain management to prevent stock-outs, and media companies similarly use real-time viewership dashboards to make on-the-fly programming decisions.
Cross-Channel Integration & Unified Profiles
Consumers engage across multiple channels and devices, so a major trend is unifying these touchpoints into one holistic view. Cross-channel (omnichannel) analytics integration is a core requirement in 2025. Brands aim to connect data from physical stores, websites, mobile apps, social media, email, and more into unified customer profiles.
This allows them to understand the complete customer journey – for example, knowing that a person who clicked a Facebook ad later purchased in-store.
Achieving this requires sophisticated data integration (often using Customer Data Platforms and identity resolution tools) and updated measurement frameworks.
In advertising, it also drives the push for deduplicated cross-media measurement.
Notably, Nielsen – long the standard for TV ratings – is retiring its panel-only measurement by Q4 2025 in favor of a Big Data + Panel hybrid to meet advertiser demand for cross-platform metrics.
This reflects how critical unified, cross-channel audience metrics have become.
Privacy, Compliance and First-Party Data
The analytics landscape in 2025 is profoundly shaped by data privacy regulations and the decline of third-party identifiers. Laws like GDPR and CCPA, along with browser changes (e.g. the phase-out of third-party cookies and Apple’s App Tracking Transparency on iOS), have limited some traditional data collection.
As a result, companies are prioritizing first-party data and privacy-compliant analytics. There’s a shift toward consent-based data collection, server-side tracking, and aggregated measurement.
Marketers list measuring ROI under new privacy constraints as a top challenge – in fact, 61% of marketers say that measuring social media ROI is their biggest challenge in 2025, partly due to attribution data loss.
In response, organizations invest in privacy-preserving analytics (techniques like data clean rooms, federated learning, and modeling) to continue gaining insights without exposing personal data. The focus on first-party data is also fueling customer loyalty programs and communities where users opt in to data sharing.
Overall, complying with privacy rules while still extracting actionable audience insights is a delicate balancing act that defines analytics strategies in 2025.
Personalization and Customer Experience Focus
Improved analytics have enabled a new level of personalization at scale. Companies can tailor content, products, and recommendations to individual preferences in a way impossible a decade ago.
Personalization is a key application of audience analytics, especially in marketing and customer experience – indeed, the “Customer Experience” application segment accounts for around 42% of the audience analytics market’s revenue in 2023.
By analyzing behavior and demographic data, brands serve personalized product suggestions, media content, emails, and app experiences.
This drives tangible results: for example, Amazon’s famous recommendation engine (powered by extensive audience data) is estimated to fuel 35% of all Amazon sales. However, companies must be cautious – there is such a thing as “over-personalization.”
A Gartner study found shoppers are 3× more likely to abandon brands that overdo personalization in a way that feels invasive. Thus, the trend is personalization with relevance and respect for user comfort, using analytics to find the right balance.
Growing Market and Competitive Solutions
Reflecting these trends, the audience analytics tools market is expanding with many solutions available. Both established tech giants and specialized startups are competing to provide analytics platforms.
We see major marketing clouds (Google Analytics, Adobe Analytics, Oracle CX Analytics, IBM Watson Customer Experience Analytics, SAS 360 etc.) refining their offerings with AI and better integration.
Simultaneously, niche players focus on specific needs – for instance, companies like Audiense specialize in social audience segmentation, NetBase Quid and Sprinklr in social listening and sentiment analytics, Quividi and Sightcorp in AI-driven visual audience measurement (e.g. analyzing retail foot traffic or digital signage viewers), and so on.
We’ll explore the competitive landscape in detail later, but suffice it to say the push for richer audience insight has created a vibrant ecosystem of analytics providers, all racing to incorporate the latest tech and to differentiate in accuracy and ease of use.
In summary, 2025’s key themes in audience analytics are about dealing with more data, from more sources, in less time – all under stricter rules – and turning those challenges into opportunities for deeper audience understanding. Next, we delve into how these trends manifest in specific sectors.
Media & Entertainment Analytics
The media and entertainment industry is undergoing rapid transformation in how it measures and analyzes audiences. Traditional TV, film, and print media have seen audience habits shift dramatically towards digital streaming, social media, and on-demand content. This has created both challenges and opportunities for analytics:
Fragmentation and Cross-Platform Measurement
In 2025, audiences consume content across linear TV, streaming services (SVOD/AVOD), social video platforms, podcasts, and more. For media companies and advertisers, measuring the total audience across these channels is a top priority.
As noted, Nielsen is rolling out Nielsen ONE, its cross-media measurement solution combining panel data with big data sources, to provide deduplicated reach across TV and digital. The company even announced it will cease its legacy panel-only TV ratings by end of 2025– a landmark end to the old model – acknowledging that advertisers demand more robust multi-source data to capture fragmented viewing.
Competing firms like Comscore are also offering cross-platform audience ratings, and new entrants (e.g. VideoAmp, iSpot) are leveraging set-top box and smart TV data to challenge Nielsen.
The end goal for all is to know true audience numbers without double counting, whether a show is watched live on cable, streamed on a phone, or clipped on TikTok.
Streaming Services and First-Party Analytics
Streaming entertainment providers (Netflix, Disney+, Amazon Prime Video, Hulu, etc.) sit on treasure troves of first-party viewer data and are extremely analytics-driven.
These platforms track every metric imaginable: content play counts, completion rates, rewatch rates, drop-off points, user search queries, etc.
They use this data not only to recommend content but to inform content production and licensing decisions.
A famous example – Netflix analyzed its audience data back in 2011–2012 and noticed a strong overlap in viewers of the actor Kevin Spacey, director David Fincher, and the political thriller genre, which gave them confidence to greenlight House of Cards (a success that validated their data-driven content strategy).
Today, Netflix’s analytics are even more advanced: reportedly 80% of the content watched on Netflix is influenced by its recommendation engine, and that engine’s personalization is credited with very low churn rates (Netflix’s churn is ~2–3%, partly thanks to keeping users continually engaged with relevant content).
Other streamers similarly analyze what content retains subscribers or drives new sign-ups. In 2025, content analytics has essentially become the “programming research” department – replacing old-school Nielsen TV sample ratings with billions of direct viewing datapoints.
For media companies, a key trend is blending these streaming insights with broader audience intel (social buzz, franchise fandom metrics, etc.) to maximize franchise value and intellectual property across platforms.
Rise of Social Video as Entertainment
A striking 2025 trend is that social media platforms have become major entertainment channels in their own right, competing with traditional studios.
Short-form video apps like TikTok, YouTube (with Shorts), Instagram Reels, and others command huge audience attention.
Deloitte observes that hyperscale social video platforms (often backed by trillion-dollar tech companies with cutting-edge AI) are now dominant forces, especially for Gen Z and Millennials.
This has two effects on analytics:
(1) Media companies need to track audience engagement on these platforms as part of their reach. A film release, for instance, is now marketed through TikTok campaigns and its success is partially gauged by TikTok views or user-generated content metrics.
(2) The platforms themselves (YouTube, TikTok) provide analytics to creators and marketers. YouTube’s Analytics dashboard or TikTok’s Creator Analytics give granular data on views, watch time, audience demographics, retention curves, etc., enabling content creators (from Hollywood studios to independent influencers) to refine their content strategy.
The competition for audience attention is fierce – traditional entertainment must measure up not just against rivals in TV or film, but against the endless stream of user-generated content.
Audience analytics in 2025 for entertainment means understanding how a teenager’s time might be split between Netflix, YouTube, Twitch, and Fortnite, for example. It’s a holistic view of “attention currency.”
Audience Engagement and Sentiment Analysis
Entertainment companies increasingly monitor engagement and sentiment around their content in real time.
Social listening tools track trending topics, mentions, and sentiment on Twitter, Reddit, and other forums to gauge audience reactions to new releases.
For instance, a sudden spike in negative sentiment after a series finale airs will be immediately flagged via analytics, prompting PR or content adjustments.
Positive engagement, like a meme from a show going viral, is quantified in impressions and engagement rate, and can inform marketing boosts.
Services like NetBase, Brandwatch, and Synthesio offer sentiment analytics that many studios and networks use to complement viewership numbers with qualitative insight.
Events like the Oscars or World Cup are accompanied by live audience analytics – measuring not just view counts but real-time social media engagement, second-screen app usage, etc., to capture the full picture of audience involvement.
Advertising Analytics in Entertainment Media
On the advertising side of media, analytics are crucial for both ad inventory yield and outcome measurement.
Broadcasters and streaming platforms use audience data to improve ad targeting and demonstrate ad performance.
Traditional TV now offers more data-driven products (addressable TV ads, for example, which serve different ads to different households during the same program, relying on audience segment data).
Streaming services with ad-supported tiers (like Netflix’s new ad tier or Hulu) provide advertisers with digital-like analytics – impressions, reach, frequency, completion rates, even brand lift studies post-campaign.
This reflects a broader industry trend: merging the granular analytics of digital advertising with the scale of television.
However, one challenge remains – attribution (connecting ad exposures to outcomes like sales) is still complex in a multi-touch world.
Media companies collaborate with advertisers on attribution models, often using third-party measurement partners or brand surveys.
Decline of Legacy Metrics, Emergence of New KPIs
As the landscape changes, some legacy audience metrics are declining in importance (or being re-imagined).
For example, linear TV ratings have been steadily dropping – in the U.S., only 49% of consumers have a traditional cable/satellite TV subscription in 2025, down from 63% three years prior– making pure Nielsen TV ratings less representative of total audience.
Meanwhile, new KPIs are coming to the fore.
Time spent is one; streamers often tout total hours viewed (Netflix regularly shares top shows by hours watched globally).
Engagement depth is another – measuring not just how many watched, but how involved they were (did they binge multiple episodes? comment on social media?).
Attention metrics are also a buzzword in advertising – companies like Adelaide or Lumen measure visual attention paid to ads, which could supplement or replace viewability metrics.
Essentially, the industry is moving from blunt measures (raw eyeballs counted) to more nuanced ones (quality of engagement, cross-platform influence, etc.), and analytics capabilities are evolving to deliver those.
In summary, the media and entertainment sector in 2025 treats audience analytics as its lifeblood.
Success is determined not just by creative intuition but by the ability to capture and interpret audience data across an ever-expanding array of channels.
The competition for audience attention is intense, and leveraging analytics – whether it’s a streamer mining viewing habits, a network combining TV and digital data, or a music label studying Spotify stats – is what separates the leaders from the laggards in entertainment.
Social Media and Digital Content Analytics
Social media platforms and digital content publishers operate in one of the richest data environments for audience analytics.
In 2025, social media usage is near all-time highs, and the interplay between audiences and content on these platforms generates real-time, highly granular data. Key trends in social media and digital content analytics include:
Massive Global Reach with High Engagement
Social media is truly global and mainstream. As of early 2025, there are approximately 5.24 billion social media user identities worldwide, representing 63.9% of the world population.
Users now spend an average of 2 hours 21 minutes per day on social platforms.
The average person uses 6.8 different social platforms per month, illustrating how audiences multi-home across Facebook, Instagram, TikTok, Twitter (X), YouTube, LinkedIn, and others.
This ubiquity means social media analytics isn’t just about marketing – it’s about understanding a large chunk of human communication and behavior.
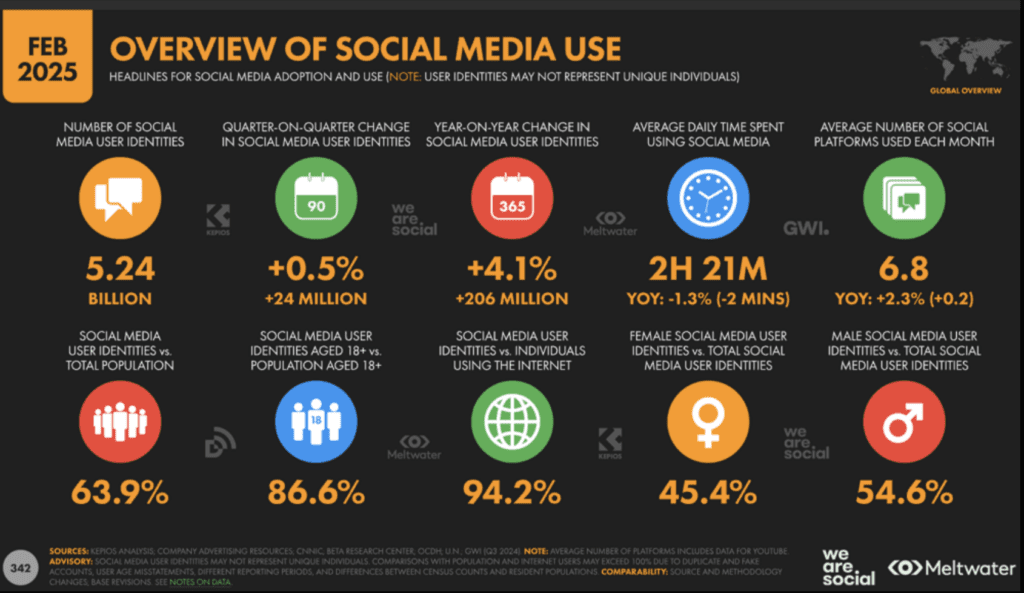
Platform Analytics for Creators and Brands
Each major social platform provides its own analytics tools to content creators and business accounts.
For instance, Facebook Insights/Meta Business Suite offers data on post reach, impressions, engagement (likes, comments, shares), audience demographics, and referral traffic for pages.
Instagram Insights similarly shows story/views metrics, follower growth, etc.
Twitter Analytics (now X Analytics) details tweet impressions, profile visits, and engagement rates.
YouTube Studio Analytics is particularly advanced, giving video creators data on watch time, audience retention graphs (where viewers drop off in a video), traffic sources, and subscriber geography.
These built-in tools allow millions of creators – from influencers to news outlets – to optimize their content strategy in near real-time.
For example, a publisher like The New York Times monitors which articles get the most social shares or referral traffic from different social networks, informing how they distribute content.
In 2025, content decisions (headlines, thumbnails, posting times) are often A/B tested and refined based on these analytics.
Social media’s algorithms reward engagement, so creators analyze what content formats (video vs image vs text) yield higher engagement and adjust accordingly.
Social Listening and Sentiment Analytics
Beyond the first-party analytics on one’s own content, there’s huge emphasis on social listening – monitoring the broader social web for conversations about brands, products, or topics.
Specialized analytics platforms like Sprinklr, Brandwatch, Meltwater, and Talkwalker aggregate public posts across social networks and use AI to gauge sentiment (positive/negative tone), identify trending themes, and even detect crises.
In 2025, companies routinely track social sentiment as a key metric for brand health.
For example, a sudden spike in negative sentiment on Twitter about a new product launch can alert a company to address issues promptly.
Social listening also helps in audience research: understanding what else your audience cares about.
Tools can tell a brand that people talking about their product also show interest in certain TV shows or hobbies, helping refine targeting (this is often called “affinity analysis”).
According to industry stats, 54% of people on social media use it to research products, so capturing those conversations is critical for marketers.
Additionally, influencer analytics has grown – tracking the reach and engagement of influencer posts and calculating media value or ROI of influencer campaigns is now a standard practice.
Advertising and ROI Measurement Challenges
Social media platforms are also advertising giants, and audience analytics is essential for campaign optimization.
Marketers leverage the granular targeting options (based on user demographics, interests, behaviors) and then analyze ad performance metrics like click-through rate (CTR), conversion rate, and cost per action.
Facebook/Meta Ads Manager and similar tools on Twitter, LinkedIn, Snapchat, etc., provide a wealth of data.
However, linking these to downstream results (sales, sign-ups) can be challenging due to privacy changes.
As noted, a majority of marketers (61%) find measuring social media ROI their biggest challenge in 2025.
This has led to evolving analytics approaches: use of UTM tracking and web analytics correlation, uptake of “conversion lift” tests (platform-provided studies to see if exposed users converted more), and modeled attribution.
Platforms like Facebook have introduced aggregated measurement and modeled conversions to cope with signal loss (e.g. after Apple’s iOS14 changes).
Despite hurdles, the spend on social media is huge – global social media ad spend was forecasted to reach roughly $220 billion in 2024 and climbing.
Thus, social analytics to measure and improve ad effectiveness remains a top priority, fueling demand for better attribution tools and creative analytics (like which ad creatives resonate most with which audience segment).
Community and Engagement Metrics
A shift in 2025 for brands on social is from just amassing followers to driving quality engagement.
Algorithms now prioritize meaningful interactions (as Facebook did with its “Meaningful Social Interactions” update).
Therefore, companies track metrics like engagement rate (engagements per impression) to gauge content effectiveness.
A smaller, engaged audience can be more valuable than a large, passive one.
Features like polls, Q&As, live streams, and stories provide new engagement data points – e.g. how many responded to a poll or questions asked during an Instagram Live – all of which feed into content strategy.
Customer service via social is another area: brands monitor response times, resolution rates, and customer satisfaction scores for interactions on Twitter or Facebook where customers often go for support.
These are often integrated into broader customer experience analytics.
Trend Analytics and Cultural Insights
Social media is the pulse of real-time culture. Analytics teams increasingly look at social data to predict trends. For instance, virality prediction: some tools attempt to predict if a piece of content is about to go viral by analyzing its early spread velocity and the networks sharing it.
Marketers also analyze hashtag trends and challenges (especially on TikTok) to jump on relevant memes in an authentic way. The extremely fast feedback loop on social means audience interests can shift overnight. A notable example: short-form video’s dominance.
Video content is king on social – posts with video gain far more reach (e.g., Facebook videos reach 135% more people organically than photo posts on average).
By 2025, brands know to look at their analytics and see the outperformers are likely video or interactive content; this drives investment in those formats.
Similarly, with the rise of social commerce, analytics might show that certain product posts drive many “save” clicks or direct checkouts (Instagram and TikTok have in-app shopping metrics), signaling a new effective sales channel.
Demographics and Niche Platform Analytics
While the overall user base is huge, analytics also reveals the differences in audience demographics and behavior by platform.
For example, a Sprout Social report on 2024 showed that Gen Z is heavily influenced by social media ads (63% of Gen Z say social ads influence their purchases) compared to older groups, and that engagement norms differ (TikTok users might engage via recreations/duets more than likes/comments).
In 2025, marketers use tools to break down their audience composition on each platform (age, gender, location data available via platform insights or surveys).
Newer or niche platforms (like Snapchat, Pinterest, or emerging ones like BeReal, etc.) each come with their own analytics quirks and limited data exportability.
Companies must decide which platforms merit focus by looking at engagement and growth analytics.
For instance, if analytics show that Pinterest drives a higher conversion rate for a retail brand than Twitter does, resources will be shifted accordingly.
The ability to compare cross-platform performance is thus valuable – leading some organizations to use unified social media management dashboards that aggregate metrics from all channels into one view for easier comparison.
Overall, social media and digital content analytics in 2025 is about leveraging the real-time, behavioral data of billions of users to inform both strategic and tactical decisions.
Whether it’s a YouTuber tweaking their content based on audience retention graphs, a brand measuring sentiment on a campaign, or a publisher optimizing headlines for shareability, the theme is using data to understand what content resonates with which audiences and why.
The platforms continue to evolve (with AI-curated feeds, new features, etc.), but a data-driven approach is the constant that helps navigate this dynamic landscape.
Retail & E-Commerce Analytics
In retail and e-commerce, audience analytics centers on understanding consumers’ shopping behaviors across both digital and physical touchpoints.
By 2025, global retail has become deeply data-driven, as retailers compete on customer experience, personalization, and operational efficiency. Key aspects of retail and e-commerce audience analytics include:
Omnichannel Customer Journey Tracking
Today’s shoppers might discover a product via a social media ad, research it on a mobile app, visit a store to see it in person, and then purchase later on a desktop website (or any zigzag combination of channels). Retailers are using analytics to stitch together these journeys.
Loyalty programs and account logins help link behavior across online and offline. Shopper ID data from in-store transactions (48% of retailers collect this) and online browsing data (57% collect) are combined to create unified customer profiles.
This allows analytics such as attribution modeling for sales (crediting the contributions of each touchpoint) and calculating lifetime value.
For instance, an omnichannel analysis might reveal that customers who engage with both the mobile app and in-store purchase have 30% higher lifetime value than those who shop through a single channel – insights that encourage strategies like buy-online-pickup-in-store (BOPIS) to move more customers into multi-channel behaviors.
Personalization and Customer Segmentation
E-commerce platforms are at the forefront of personalization.
By analyzing clickstream data, past purchases, and even media preferences (26% of retailers look at customers’ media/entertainment interests) to inform offerings, retailers segment audiences and tailor experiences.
For example, 74% of e-commerce companies have some form of website personalization program in place, whether it’s recommending products “You May Also Like,” sending personalized email offers, or reordering the homepage based on user profile.
Machine learning models segment shoppers into clusters (e.g., bargain hunters, brand loyalists, high-spenders, lapsed customers) and content is customized accordingly.
Amazon is a prime case – its homepage is unique to each customer, showing products and deals based on that individual’s browsing and buying history. The payoff is significant: personalized product recommendations are credited with a substantial portion of Amazon’s revenue (as noted, ~35%).
Retailers track CX metrics like customer satisfaction (through surveys), NPS, and engagement time on site/app.
They correlate these with behavioral data: e.g., linking a drop in satisfaction scores to specific pain points in the shopping journey (maybe a slow website section or confusing checkout step), then using analytics to test improvements.
AI-Powered Demand Forecasting & Merchandising
A critical application of analytics in retail is forecasting demand and optimizing inventory – essentially aligning audience interest with supply.
AI-driven models analyze historical sales, search trends, social media sentiment, even weather and local events, to predict what products will be in demand where and when.
This directly ties to audience analytics: for instance, a spike in online searches or social mentions for a certain sneaker can prompt a retailer to stock up in regions where that interest is highest.
Merchandising teams use data dashboards that highlight fast-rising products (based on pageviews, add-to-cart rates, etc.) so they can respond quickly.
On the flip side, slow-moving inventory is flagged by analytics, and retailers might enact markdowns or targeted promotions at segments likely to buy those items (determined by past affinity).
Real-time inventory analytics also ensures popular products found via online channels are actually in stock – nothing loses a customer faster than clicking an ad only to find the item unavailable.
Many retailers now integrate their e-commerce analytics with in-store systems: if an item is trending online in a city, local stores can be alerted to feature that item more prominently (a practice some call “digital pulse to store action”).
In-Store Analytics and Offline Data
Brick-and-mortar retail isn’t left out – it’s becoming as data-rich as online. Technologies like smart shelves, RFID tags, video analytics, and beacon sensors provide insight into physical shopper behavior.
Retailers measure things like in-store dwell time, traffic flow, and product interactions.
About 42% of retailers collect data on in-store movement and behavior (e.g., tracking how customers navigate aisles).
This informs store layout optimization – analytics might show that certain displays attract a lot of stopping customers but don’t convert to sales, prompting a layout change or better on-shelf info.
Camera-based analytics (with privacy safeguards like anonymization) can count how many people pass by a window display versus enter the store, giving a “conversion rate” for storefronts.
Heatmaps of a store can be generated to see which areas are most frequented. Retailers also heavily analyze point-of-sale transaction data: not just what sells, but basket analysis (which items are bought together).
Combined with loyalty data, they can see segment patterns (e.g., young urban professionals shopping on weeknights have a different basket mix than suburban families on weekends). All this offline data is merged with online profiles when possible to enrich the single customer view.
E-Commerce Funnel and UX Analytics
On e-commerce websites and apps, analytics focus on the conversion funnel and user experience. Metrics like product page views, add-to-cart rate, checkout completion rate, and bounce rate at each step are closely watched.
The average shopping cart abandonment rate is around 70% in online retail, so analyzing where and why users drop off is crucial.
Retailers run experiments (A/B testing) constantly: testing different page designs, checkout processes, or recommendation algorithms and measuring impact on conversion or average order value.
Tools like Google Analytics (GA4) or Adobe Analytics track user flows and can segment behavior by traffic source or campaign.
If a particular ad campaign brings high traffic but those users have an 80% bounce rate, analytics will reveal that disconnect and marketers can adjust targeting or landing pages.
Site speed and technical performance analytics also feed into UX – slow page loads can kill conversions, so tracking load times and correlating with user drop-offs is standard practice now.
Many companies employ session replay and clickstream analysis (via tools like Contentsquare or Hotjar) to literally see how users interact – for example, seeing that many users hover over an unclear icon might indicate a UX issue.
By 2025, e-commerce leaders treat UX analytics as a continuous feedback loop to refine the shopping experience.
Mobile Commerce and App Analytics
Mobile deserves special mention as it’s dominating online retail. As of mid-2020s, about 65% of e-commerce site visits globally come from mobile devices, and over 50% of online transactions are now on mobile.
In the US, mobile commerce sales are projected to reach $710 billion in 2025, making up 44%+ of all US e-commerce sales.
Retailers therefore invest heavily in mobile app and mobile web analytics.
They track mobile-specific metrics such as app launch frequency, retention rate, cohort analysis (how many users continue to use the app after X days), and in-app engagement (product views, wishlist additions, etc.).
A known challenge is mobile conversion rates tend to be lower than desktop (globally ~2.3% on mobile vs 3.8% on desktop), so analytics are used to diagnose friction points on small screens and to design mobile-friendly experiences.
Many retailers also embrace mobile wallets and payments – with a forecast 33% growth in mobile payments worldwide and trillions in transaction value– meaning they analyze usage of Apple Pay, Google Pay, etc., in their checkout flow.
Additionally, social commerce (users buying directly through social media apps) is rising – e.g., 53% of Americans shop on social media weekly– so retailers track referral traffic and conversions coming from social platforms and even integrate shops within those platforms (like Instagram Shops), which then offer their own analytics dashboards.
Customer Retention and CLV Analytics
In retail, it’s not just about acquiring customers, but retaining them.
Audience analytics is applied to loyalty and CRM (Customer Relationship Management) to maximize Customer Lifetime Value (CLV).
Retailers segment customers by RFM (Recency, Frequency, Monetary value) and identify VIPs versus at-risk customers.
Analytics might show, for example, that 20% of customers drive 50% of revenue, leading to special retention campaigns for that 20%.
Churn modeling is used to predict which customers are unlikely to return – for instance, if someone hasn’t made a purchase in 6 months and has low site engagement, they get flagged for a win-back campaign (perhaps a personalized discount).
The effects of those campaigns are then measured (response rate, incremental revenue).
With subscription-based retail models (like subscription boxes or Amazon Prime memberships), cohort analytics and churn rates are vital metrics.
Marketing mix modeling and attribution also come into play to understand how different channels (search ads, social ads, email, etc.) work together to drive sales, guiding budget allocation.
Retail Media Networks and Ad Analytics
A very hot trend by 2025 is retailers themselves becoming media publishers via retail media networks – essentially selling ad placements on their own platforms (websites, apps, in-store displays) using their first-party shopper data to target ads.
Retail giants like Amazon, Walmart, Target, and grocery chains have built significant advertising businesses.
Analytics is the backbone of these: retailers provide brands with reports on ad impressions, click-throughs, and conversion within their ecosystem.
They can show, for example, that an ad for cereal on their site got X impressions from health-conscious shoppers and drove Y online sales plus Z in-store sales (using loyalty card data to attribute in-store purchases to seeing the ad online – a unique capability of retail media).
The retail media market is booming (with global spend expected to reach into the tens of billions).
For advertisers (CPG brands, etc.), the appeal is the rich audience analytics: they can advertise to “beauty enthusiasts who buy organic” or “frequent snack buyers” based on actual purchase data, and then get detailed analytics on performance.
This is a new frontier where audience analytics meets advertising within the retail context, and it’s likely to be a major growth area for analytics innovation.
Macro Trends Reflected in Analytics
Broader consumer trends (like shifts due to the pandemic or economic changes) are also evidenced in retail analytics.
For instance, the pandemic spike in online shopping was captured in analytics: U.S. click-and-collect sales jumped 60.4% in 2020 due to safety concerns, and even in 2025 retailers watch e-commerce vs. store split closely as habits normalize.
Sustainability is another trend – more consumers care about brand values, so some retailers now track and segment “eco-conscious customers” (perhaps identified via surveys or purchase of sustainable products) and analyze their behavior separately.
The rise of direct-to-consumer (D2C) brands means a lot of smaller companies are heavily reliant on digital analytics from day one to refine their product-market fit and growth marketing.
In essence, retail analytics not only responds to trends but helps detect them early: e.g., noticing a category’s sales climbing unusually might indicate a new lifestyle trend among customers.
In conclusion, retail and e-commerce analytics in 2025 is incredibly multi-faceted, covering everything from granular on-site user behavior to high-level strategic forecasting.
Retailers who effectively harness these analytics can optimize inventory, delight customers with personalized experiences, and run more efficient marketing – all of which are necessary in a very competitive retail environment.
Those that don’t leverage their audience data risk falling behind, as consumers now expect retailers to “know them” and meet their needs seamlessly across channels.
Events and Live Experiences Analytics
The events industry – spanning conferences, trade shows, concerts, sports, and even virtual webinars – has become much more data-driven in recent years.
After the shock of 2020–2021 (when many events went virtual), 2025 finds the events landscape embracing both in-person and hybrid formats, each providing opportunities for rich audience analytics.
Key trends and practices in event analytics include:
Attendee Engagement Tracking
Audience engagement is now the premier metric for event success.
In fact, over 82% of B2B marketers consider attendee engagement a key KPI for determining an event’s impact.
This goes beyond simple attendance numbers – it’s about how attendees interact, for how long, and how deeply.
Event organizers use mobile event apps or interactive platforms that track attendee actions: sessions favorited, live poll responses, questions asked during Q&A, networking connections made, booth visits (scanned via RFID or QR codes), etc.
All these provide a quantitative view of engagement. For example, a conference app might show that 75% of attendees created a personalized agenda (which is a positive engagement signal), or that Session A had 200 chat messages posted while Session B had 20 (indicating A was more engaging or controversial).
These insights help planners identify what content resonated. Post-event surveys are another piece – often integrated into apps – providing qualitative feedback that can be quantified (e.g., session ratings, speaker ratings).
The focus on engagement is driven by recognition that a passive audience isn’t deriving full value; engaged attendees are more likely to return and to achieve the event’s goals (learning, networking, buying, etc.).
Organizers now often produce engagement reports for internal stakeholders or event sponsors, summarizing how attendees participated.
Virtual and Hybrid Event Analytics
Virtual components of events (which became mainstream during the pandemic) bring web-like analytics to the events world.
Every click and view can be tracked in a virtual event platform.
Metrics such as number of logins, average viewing time per session, chat activity, and resource downloads are standard.
Hybrid events (with both live and virtual audiences) yield a combination of in-person and online data.
One challenge is maintaining virtual attendee engagement – and indeed 67% of event marketers say it’s challenging to keep virtual attendees engaged.
Analytics can highlight drop-off points in a virtual session (maybe the presentation ran too long and viewers left) or identify that virtual attendees never visited the expo booths section of the platform, prompting a rethink of virtual expo design.
Organizers also compare engagement between in-person and virtual audiences.
For instance, they might find virtual attendees ask 2x more questions (perhaps because of the anonymity or ease of typing questions) – an insight that could encourage hybrid formats to leverage the virtual audience for Q&A more.
Additionally, on-demand viewership (people watching session recordings after the live event) is tracked, extending the engagement window.
Some events report that a significant portion of total views occur post-live, which affects how they package content and keep the community discussion going after the event.
Attendee Journey and Behavior Insights
Similar to customer journey analytics in retail, events are analyzing the attendee journey: from registration to check-in to session selection to post-event actions.
Registration analytics track when and how people register (e.g., a growing trend of last-minute registrations, which 63% of event marketers cite as a challenge).
This informs marketing timelines – if data shows most sign-ups happen within 2 weeks of the event, organizers might concentrate promotional spend in that window.
On-site behavior is tracked via technologies like NFC badges or wearable beacons that detect when an attendee enters a session room or visits a sponsor booth.
This can produce a “digital footprint” for each attendee (e.g., John Doe attended 5 sessions, visited 3 exhibit booths, spent total 6 hours on site, and interacted with X features in the app).
Aggregating these, organizers see overall popular sessions, average sessions per attendee, dwell times, etc. If one particular track has low attendance, that’s valuable feedback for future content planning.
Flow analytics can even be done: understanding how attendees move through a venue.
Large trade shows often use heatmap analytics to see which halls got the most traffic or which times of day had peaks, helping with layout decisions and crowd management (important for safety as well as for sponsor ROI).
ROI for Exhibitors and Sponsors
For events that involve exhibitors (like trade show booths) or sponsors (brands paying to sponsor sessions, lobbies, etc.), providing analytics to prove ROI has become essential.
Rather than just saying “10,000 people attended the trade show,” organizers now supply data like number of leads captured at each booth, average time spent at booth, number of brochure downloads from virtual booth, and so on.
Many events equip booths with lead scanning apps – when an attendee allows their badge to be scanned, it logs a lead.
Exhibitors can get a report: e.g., 150 leads scanned, with breakdown by attendee industry or interest (if that data is in the registration info).
They may also get qualitative analytics like poll responses from attendees who visited their booth or traffic patterns around their booth area.
Sponsors for sessions get metrics on how many viewed those sessions (both live and on-demand), engagement during the session, and any direct interactions (perhaps a sponsor-provided resource download count).
The sponsorship segment in B2B events is projected to grow at ~12% CAGR (2021–2028), and with 33% of businesses dedicating 21%+ of their event budgets to sponsorships, the accountability via analytics is crucial.
A notable stat: 49% of marketers say audience engagement is the biggest factor for a successful event, which ties into sponsorship – engaged audiences are more valuable to sponsors.
Thus, events with high engagement metrics can command higher sponsorship fees, backed by data.
Real-Time Adjustments and Personalization
During events, organizers increasingly make real-time adjustments based on data – something that was rare in the past.
For instance, if analytics show a particular breakout session is over-capacity (room full, with many trying to get in), they might repeat that session later or move it to a larger room on the fly if possible.
If a live poll indicates most attendees are interested in a sub-topic, a panel might spend more time on it.
Some events use second-by-second feedback (through live sentiment polling) to gauge how content is landing and tweak the discussion.
Moreover, events are experimenting with personalized experiences via analytics: event apps might recommend sessions or people to network with based on your profile and behavior (similar to content recommendations).
If the app knows you favor workshops over lectures and you showed interest in AI topics, it might suggest “Workshop on AI at 2pm” to you.
These recommendation algorithms rely on clustering attendees by interest – essentially an application of audience analytics to the event content itself.
Post-Event Analytics and Continuous Improvement
Once the event concludes, the analytics work isn’t over.
Post-event, organizers compile an event report combining all data: registration vs attendance numbers, engagement stats, feedback survey results, lead stats for exhibitors, social media mentions and reach (since many events spur a social conversation which can be tracked via hashtags).
They look at ROI metrics:
For internal events, it might be employee training outcomes;
for marketing events, it might be pipeline generated.
Notably, 86% of B2B organizations report positive ROI within 7 months of holding a hybrid event, indicating that events do pay off and analytics helps demonstrate that by tracing leads to deals.
Another stat: 70% of event professionals say media exposure generated is a top metric for event ROI– thus they analyze press coverage, social reach, and content downloads attributable to the event.
However, 19% of companies still struggle to quantify event ROI, often due to data silos or lack of tracking (highlighting room for improvement in analytics integration with CRM and sales systems).
Event teams use the collected data to iterate – e.g., if feedback says content was great but networking opportunities were lacking, they might add structured networking sessions next time and then measure if networking metrics improve (like number of connections made or meeting RSVPs in the app).
Experience and Satisfaction Metrics
Events are inherently experiential, so analytics also cover attendee satisfaction and experience quality. Via post-event surveys or even live NPS prompts, organizers gauge satisfaction levels.
In 2025, 87% of event planners actively strive to incorporate Diversity, Equity, and Inclusion (DE&I) into events– one can measure progress on this by analyzing attendee demographics vs. engagement (e.g., did all groups report similar satisfaction? Did diverse voices participate in Q&A?).
Another modern consideration: health and safety analytics (made prominent by COVID) – tracking things like crowd density, ventilation metrics, or health survey responses, though by 2025 this might be less front-of-mind than it was in 2021.
For large consumer events like music festivals or theme parks, they even look at things like emotion analysis via cameras or social posts (e.g., are people smiling in photos), though that’s still emerging and can raise privacy concerns.
In summary, events analytics in 2025 transforms events from one-off gatherings into data-rich, optimizable experiences.
Organizers and stakeholders demand proof of value, and analytics delivers it – from demonstrating attendee engagement to capturing leads and informing better content for next time.
The mindset has shifted such that an event is not over when the doors close; it lives on through data analysis, follow-ups, and insights that feed into the next cycle of planning.
The competitive advantage lies with event organizers who can leverage these insights to continuously improve the attendee experience and event ROI.
Mobile Apps & Gaming Analytics
Mobile apps – including everything from social apps to gaming, productivity, and retail apps – generate an enormous amount of user behavior data.
In 2025, mobile usage is dominant in digital life, and thus mobile app analytics is crucial for understanding audiences and improving mobile user experiences.
Similarly, gaming (much of it mobile gaming) is a huge industry driven by analytics. Key points in mobile apps and gaming analytics:
Mobile Dominance and Usage Patterns
The sheer scale of mobile is our starting point: There are over 6.3 billion smartphone users worldwide, and mobile apps account for ~70% of all US digital media time spent by users.
The average smartphone owner uses about 10 apps per day and 30 apps per month, indicating both heavy usage and a competitive attention economy (apps vie to be in those top 10 used daily).
For app developers and businesses, this means analytics must focus on retention and engagement – how to be one of those apps people keep coming back to. Key metrics include Daily Active Users (DAU), Monthly Active Users (MAU), and engagement time.
A critical compound metric is stickiness (DAU/MAU), which indicates what fraction of monthly users use the app daily.
In 2025, many apps aspire to the stickiness seen in social or messaging apps (which can have 50%+ DAU/MAU).
If an app’s analytics show a stickiness of, say, 10%, that’s a sign that users aren’t forming a habit, and product teams will investigate why (perhaps the app’s value proposition is more occasional use, or there are onboarding issues).
Onboarding and Funnel Analytics
For any mobile app, the user onboarding process (from install to sign-up to first key action) is critical. Analytics helps pinpoint where users drop off.
For instance, if 100,000 people install an app, but only 50,000 open it and 20,000 complete account creation, there’s a funnel drop-off that can be analyzed step-by-step.
Tools like Firebase Analytics, Mixpanel, or Flurry allow creation of funnels to see conversion rates at each step.
Perhaps the data shows 30% drop off on the “permissions request” screen – a cue to redesign how permissions are asked.
By improving these conversion rates, apps can dramatically increase their activated user base without additional marketing spend.
In 2025, it’s common for apps to run A/B tests on onboarding flows guided by analytics (for example, testing a shorter tutorial vs. an interactive guide, and measuring which yields higher 7-day retention).
Retention and Cohort Analysis
Retention is king for apps.
Analytics platforms offer cohort analysis, which tracks how different groups of users behave over time since installation.
For example, one might look at the cohort of users who installed in January and see what percentage are still active 1 week, 1 month, 3 months later, then compare to the February cohort.
If later cohorts retain better, it could indicate improvements in the app or better user acquisition targeting.
Industry benchmarks show that retention drops off quickly for many apps – by day 30, perhaps only 10-20% of users still use an average app (one stat indicated Android apps retain only ~2.1% of users by day 30 in worst cases, though that sounds like an extreme low average for broad set of apps).
The goal of analytics-driven growth teams is to flatten that churn curve.
They identify what factors correlate with higher retention – e.g., in a music app, users who create a playlist in their first session might be far more likely to stick around.
So product managers might design the onboarding to encourage playlist creation, then use analytics to see if that intervention indeed boosted retention of the next cohort.
Feature Usage and Engagement Depth
Mobile app analytics goes deep into feature usage.
Product teams want to know which features are used frequently and which are ignored.
For example, a messaging app might track how many users try voice messages or video calls. If only 5% of users use a particular tab or section of the app, they might consider removing or revamping it.
Time-spent on each section, or sequences of actions (path analysis) can reveal common usage patterns.
For instance, analytics might show that 80% of users who use a weather app just check the current weather and leave, whereas only 20% click on the radar map – this insight can guide simplifying the UI or focusing on the core use case.
Many teams set up event tracking for every important user action (click “Buy”, “Share Post”, “Complete Level”, etc.) and then analyze frequency and correlations.
If an e-commerce app sees a lot of “Add to wishlist” but not enough purchases, they might introduce a promotion targeting wishlist items.
If a fitness app notices users often drop off after completing 3 workouts, they might add motivational notifications or new content around that time.
Monetization and Conversion Analytics
Apps often have revenue models – whether in-app purchases, subscriptions, or ad monetization.
Conversion analytics focuses on how users move down the monetization funnel. For a subscription app, that might be the free trial start -> subscription conversion.
Metrics like conversion rate, trial retention (how many continue after trial), churn rate, and LTV (lifetime value) are computed.
Pricing experiments are done and analyzed for impact.
In free-to-use ad-supported apps, the focus might be on ad engagement metrics and balancing them with user experience (e.g., how showing ads affects retention).
In gaming, monetization analytics is extremely sophisticated: game companies track the spend behavior of players, identifying whales (the small % of players who contribute the majority of revenue) vs. average spenders vs. free players.
They analyze what triggers spending – maybe a certain level of difficulty or a sale on in-game currency – and optimize those triggers.
For example, analytics could show that players are likely to make their first purchase when they hit a difficulty wall at level 5, so the game might ensure a compelling offer is presented at that point.
A/B testing is heavily used in games: different segments of players might see different in-game events or store layouts, and their spending and retention are compared through analytics.
Gaming Analytics and Player Behavior
In gaming (especially mobile games which are often free-to-play), analytics essentially drives the ongoing game design post-launch. Developers track level completion rates, win/loss ratios, session length, and social interactions.
If a level in a game has only a 40% completion rate and that’s causing players to quit, designers will know via analytics and adjust difficulty in an update.
Social or competitive features (like leaderboards) also provide analytics – do they increase retention?
Are players who join guilds/clans more engaged (often yes, and thus games encourage social play)? Esports and live-service games track concurrent users, peak times, and engagement during in-game events (which in turn influences scheduling of events).
One modern angle is user-generated content analytics in games that allow creation (like Roblox or Fortnite’s creative mode) – tracking which user-made experiences are popular to possibly promote or emulate them.
Performance and Crash Analytics
Part of user experience analytics is technical performance. Tools like Firebase Crashlytics or Sentry track app crashes and errors.
High crash rates or bugs can drive users away, so engineering teams monitor these analytics constantly, often setting thresholds (e.g., “crashes affecting >2% of sessions must be fixed immediately”).
They also look at device-specific analytics – if the app performs poorly on certain phone models or OS versions, that’s actionable.
Additionally, app store analytics (from Apple App Store and Google Play Console) provide data on installs, uninstalls, rankings, reviews, etc. An uptick in uninstalls after a new release might signal a problem introduced, which internal analytics can help diagnose.
User Feedback and Sentiment (Reviews, etc.)
App ratings and reviews are a valuable qualitative data source which can be quantified in some ways.
Many companies perform sentiment analysis on app reviews to categorize common complaints or praise (e.g., 30% of recent reviews mention “login issue” – a flag to fix that).
They correlate release changes with review trends to see if updates improved sentiment. Some also integrate in-app surveys or NPS prompts at key moments, feeding that data into overall product analytics to prioritize features.
AI and Personalization in Apps
By 2025, many apps have AI-driven personalization internally – and they use analytics to fine-tune those algorithms.
A news app, for example, analyzes which recommended articles get clicked to continuously improve its recommendation model for each user.
As another example, 63% of mobile app developers plan to implement AI features by 2025, indicating features like chatbots, predictive suggestions, etc.
These AI components themselves are evaluated through analytics – how often does the AI chatbot successfully resolve an issue?
Does the personalized feed increase session length? This merges product analytics with machine learning metrics.
Privacy and Data Limitations
It’s worth noting that mobile analytics has had to adapt to privacy changes.
Apple’s App Tracking Transparency (ATT) introduced in 2021 means users must opt-in to cross-app tracking. This drastically reduced the data available for user acquisition attribution (identifying which ad or source led to an install).
By 2025, marketers have adjusted by using aggregate analytics (SKAdNetwork on iOS provides campaign-level performance but not user-level data) and focusing more on on-platform metrics.
For example, instead of granular tracking of every ad to in-app action for every user, advertisers look at blended metrics: if we spent X on Facebook Ads, did our overall installs and revenue lift accordingly in that period? It’s a more modeled approach.
First-party analytics (within the app) remain rich, but linking a specific user’s outside behavior is harder.
Also, compliance with privacy means collecting only necessary data and being transparent – many apps now show users a dashboard of what data is collected and how it’s used, as per regulations.
This environment pushes app analytics teams to be savvy in gleaning insights while respecting user consent choices and using aggregated data when needed.
In summary, mobile app and gaming analytics in 2025 is about optimizing the user lifecycle – from acquisition to engagement to monetization – using data.
The competition in app stores (millions of apps available) means that only apps which intelligently improve and cater to their audiences will thrive.
Analytics provides the evidence for making product decisions: adding features that users want, removing friction, and finding the levers that increase satisfaction and revenue.
Gaming, being a particularly data-savvy sector, often leads the way in real-time analytics experimentation and monetization techniques that later spread to other app categories.
As mobile devices continue to be the primary personal computing platform for most of the world, the importance of mastering mobile audience analytics cannot be overstated.
Leading Companies and Case Studies in Audience Analytics
To illustrate these trends in practice, let’s look at case studies of leading companies and platforms that excel in using audience analytics, as well as highlight major providers in the analytics landscape:
Netflix (Entertainment/Streaming)
Netflix is often cited as a gold standard for data-driven strategy. The company analyzes data from its 238+ million subscribers to inform everything from content recommendations to production.
As mentioned, Netflix’s personalized recommendation engine drives roughly 80% of viewing hours on the platform, making the vast content library accessible and tailored to individual tastes.
The impact of this audience analytics is massive: it’s credited with keeping churn extremely low (Netflix retains members at rates far higher than cable TV), and Netflix itself said that its recommendation algorithms save it over $1 billion per year by preventing cancellations.
On the content side, Netflix uses audience analytics to decide which shows to invest in, which to cancel, and how to market them.
They famously used analytics to guide the creation of House of Cards.
More recently, they might look at how many viewers start but don’t finish a new series – a signal of poor engagement – or which scenes in a stand-up special get rewatched the most – which could inform future comedy content.
Netflix also A/B tests relentlessly: everything from the artwork thumbnails shown for each title (personalized based on your viewing history) to the timing of auto-playing previews is tested with segments of users and measured.
This culture of analytics-backed experimentation is a big part of Netflix’s innovation engine.
Key takeaway
Netflix’s success demonstrates how deep audience analytics (at scale) can directly translate to business outcomes in media – higher engagement, better content ROI, and customer retention.
Amazon (Retail/E-commerce)
Amazon is another prime example of audience analytics mastery.
It has a multifaceted use of analytics across retail and its digital services.
The product recommendation engine on Amazon’s site (the “Customers who bought X also bought Y” and “Recommended for you” sections) is estimated to generate 35% of Amazon’s e-commerce revenue, highlighting how effective personalized suggestions based on browsing and purchase history can drive sales.
Amazon analyzes every customer click and purchase.
They use this data for personalization (homepage, emails), for inventory forecasting (their supply chain is finely tuned with demand predictions per region), and for dynamic pricing (prices on Amazon can change frequently based on demand, competitor pricing, and customer behavior patterns).
Another area is Amazon’s massive advertising business (Amazon Ads).
Advertisers selling on Amazon get analytics dashboards showing the performance of their ads (impressions, click-through, sales attributed).
Amazon uses conversion data to help advertisers target better (for example, showing which search keywords lead to more sales of their product).
Case in point
Amazon’s Prime Day events are data-driven marathons – they analyze what categories and deals spur the most engagement and continually refine the event.
Through Prime and its streaming service (Prime Video), Amazon even connects content analytics to commerce – e.g., analyzing if watching a cooking show leads to increased purchases of certain kitchen products.
Additionally, Amazon Web Services (AWS) provides analytics tools (like QuickSight, etc.) and machine learning that other companies use, meaning Amazon is also a provider enabling others to do audience analytics.
Key takeaway
Amazon’s ability to recommend the right product, at the right time, to the right customer – and ensure it’s in stock and delivered fast – is fundamentally powered by their advanced audience and operational analytics.
Meta / Facebook (Social Media Advertising)
Facebook (now Meta) built one of the most sophisticated audience analytics and targeting engines in advertising history. Pre-2020,
Facebook’s algorithms had nearly unfettered access to user behavior across the web and apps, allowing them to micro-target ads and measure conversions. Changes like iOS14’s privacy features have curbed that a bit, but Meta still handles an enormous amount of data.
The Facebook Ads Manager uses audience analytics to let advertisers define custom audiences (e.g., people who visited their website in the last 30 days, or lookalikes of their customers) and then provides analytics on ad performance and conversion. On the user side, Meta’s feed algorithms (for Facebook, Instagram) analyze each user’s engagement with posts, time spent on content, etc., to personalize the feed.
One interesting adaptation is Meta’s increasing use of AI recommendation (showing you Reels or posts from accounts you don’t follow if they seem to match your interests, an approach inspired by TikTok). They would have tested this via analytics showing such recommendations can increase user time spent.
Case study example
In response to ATT reducing individual-level tracking, Meta deployed aggregated analytics approaches like conversion modeling to estimate how many conversions an ad likely drove even if it can’t observe all of them.
They also encourage businesses to use Conversions API (sending events directly from server to Facebook) to recover some lost signal.
These are technical shifts, but fundamentally driven by the need to keep feeding the analytics models with enough data to optimize ads.
On the user metrics front, Facebook’s own reporting in late 2024 indicated user growth in certain regions stagnating – their analytics likely pushed them to diversify (hence the push into the metaverse and VR with Oculus, trying to create new platforms to gather audience engagement).
Key takeaway
Meta’s business thrives or fails based on its analytics of audience behavior for ad targeting; it showcases the power and also the new challenges of analytics in a more privacy-conscious world.
Google (Digital Analytics & AdTech)
Google is both a user of audience analytics and a provider of analytics tools.
As a user: Google’s products like Search, YouTube, and Android all rely on analytics.
YouTube, for instance, uses audience analytics to recommend videos (leading to those multi-hour watch sessions many experience) and to surface trends.
It tracks video performance (views, watch time, likes/dislikes, shares) and creator behavior to constantly tweak its algorithm.
As a provider: Google Analytics (GA) has been the most widely used web analytics platform, installed on well over half of all websites.
In 2025, Google has transitioned users to Google Analytics 4 (GA4), which is designed for a cookieless future with an event-based model and built-in machine learning (to plug gaps in data with modeled insights).
GA4 provides businesses with cross-platform analytics (web + app), allowing them to see unduplicated users across their site and Android/iOS apps, for example.
Google also offers Google Ads and AdSense analytics, giving advertisers and publishers insights into audience segments, ad viewability, etc. Google’s strength has been connecting its analytics to action – e.g., you can create an audience in Google Analytics (say, users who viewed product pages but didn’t purchase) and directly export that to Google Ads for retargeting campaigns.
Another case: Google’s search ads use a Quality Score system partly based on user behavior analytics (click-through rates of ads, landing page experience as measured by bounce rate or speed).
Internally, Google’s search algorithms continuously analyze what users click (and how quickly they return to search results, i.e., did the result satisfy them or not) to refine rankings – essentially treating each search as an experiment.
Key takeaway
Google’s entire ecosystem, from search to advertising, is built on collecting user interaction data and using it to improve relevance.
As a dominant analytics tool provider, they also influence how millions of businesses measure audiences. Their shift to GA4 and privacy-centric modeling indicates where analytics is heading for everyone.
Adobe (Marketing Analytics Suite)
Adobe might not be as household a name to consumers in this space, but in the enterprise, Adobe Analytics (part of Adobe Experience Cloud) is a leading platform for audience analytics, often used by large companies for complex web/app analytics needs.
Adobe’s tools allow deep customization and integration (with data from CRM, etc.), and they position themselves as a solution for real-time customer profiles and journeys.
A case study: a major media company might use Adobe Analytics to track user behavior on their streaming app, tie it with Adobe Audience Manager (a data management platform) to create segments for ad targeting, and use Adobe Target to A/B test experience variations – all within Adobe’s ecosystem.
Adobe’s acquisition of Omniture in 2009 and subsequent growth means they power analytics for many top ecommerce and media sites.
They emphasize customer journey analytics, able to follow a user from, say, an email click to website browse to mobile app purchase.
Adobe also incorporates AI (Adobe Sensei) to find anomalies or predict churn.
For example, Sensei might flag that a certain segment is converting much less this week than usual – a prompt for marketers to investigate.
Key takeaway
Adobe’s presence shows the enterprise demand for integrated analytics solutions that handle online/offline and at scale, beyond what Google’s free tool offers.
It’s a competitive landscape including Oracle, IBM, and SAP in various capacities, all catering to large organizations that need robust audience analytics and are willing to invest significantly for it.
SAS and IBM (Enterprise Analytics)
SAS and IBM have provided analytics solutions (like SAS Customer Intelligence 360 or IBM Watson Analytics for Customer Experience) often used in sectors like finance, telecom, and healthcare where extremely advanced analysis and sometimes on-premises data due to security are needed.
They might be used for heavy data mining – for instance, a bank analyzing transactional data alongside web behavior to detect which customers are likely to churn or buy a new product.
IBM Watson has been applied in marketing to do things like cognitive analysis of customer feedback or predictive segmentation.
While not as visible in the marketing blogosphere, these tools underscore that audience analytics isn’t only about web clicks – it extends to call center interactions, ATM visits, or any touchpoint, and companies like SAS and IBM have specialized in bringing those together with statistical rigor.
A telecom case study might involve SAS analyzing mobile usage data plus app interactions to figure out which customers should get an upsell offer for a higher data plan – a very data-heavy and proprietary data scenario.
Nielsen and Comscore (Audience Measurement Firms)
We discussed Nielsen’s transformation earlier; it’s historically measured TV and radio audiences (panel-based) and is moving into integrating set-top box data, smart TV data, and digital.
Nielsen One aims to be the standard for cross-media reach.
Meanwhile, Comscore measures digital audiences (via panels and tag-based tracking on sites/apps) and provides products like Comscore Media Metrix (ranking websites by audience size) and Comscore Campaign Ratings.
A case study with Comscore: a large publisher might use Comscore to understand their de-duplicated audience reach across their website and their YouTube channel and Snapchat Discover presence, for example, which advertisers want to know.
Nielsen is also branching into outcome-based analytics (tying exposure to purchase, via partnerships with credit card data etc.).
These firms show that there’s a whole segment of the industry focused on independent third-party audience analytics, crucial for advertising trade (where both sides need a trusted number).
They face competition from tech-driven analytics startups and evolving privacy regulations but remain key players.
Specialized Analytics Startups and Tools
There are many niche players tackling specific analytics challenges:
Mixpanel, Amplitude – focused on product analytics for apps, giving very granular funnel and retention analysis. Tech companies (e.g., Uber, Spotify historically) have used these to fuel rapid product iteration.
Sprout Social, Hootsuite, Brandwatch – focused on social media analytics and management, as mentioned, helping brands dissect their social audience engagement.
Qualtrics, Medallia – dealing with experience analytics, marrying survey feedback with behavioral data.
CDPs (Customer Data Platforms) like Segment, mParticle – not analytics per se, but enabling unified audience data, which then analytics can be layered on.
Tableau, Power BI – data visualization tools that companies use to build custom dashboards for audience metrics.
Quividi, IntelliVision – providing analytics for physical spaces using computer vision (e.g., a digital billboard can measure how many people looked at it and for how long, and even approximate demographics – this is audience analytics for out-of-home advertising).
Localytics, Leanplum (now CleverTap) – mobile engagement analytics and messaging, focusing on using analytics to drive personalized push notifications and in-app campaigns.
Each of these caters to different “puzzle pieces” of the broader audience analytics needs of organizations.
The competitive landscape in 2025 is thus one where the major players (Adobe, Google, Oracle, SAS, IBM, etc.) offer end-to-end suites, while numerous innovative companies offer point solutions or specialized capabilities.
Many large organizations use a combination: perhaps Google Analytics for basic web metrics, Mixpanel for detailed product analytics, Sprinklr for social listening, and an internal data warehouse where they compile it all for their data science team to analyze.
An emerging trend is the desire to consolidate these if possible – hence the appeal of integrated platforms – but flexibility and depth of insight are also important, so best-of-breed solutions continue to thrive.
Future Outlook and Conclusion
The future of audience analytics beyond 2025 looks both promising and challenging, with several trajectories already visible:
Even More AI-Driven Insights
AI and machine learning will further penetrate analytics – moving from assisting analysis to largely automating it.
We can expect more “auto-generated insights” where the system not only visualizes data but tells you in natural language what’s happening (“Insight: Users from region X showed a 15% increase in conversion this week, likely due to…”) and even recommends actions (“Consider increasing budget for campaign Y in region X”).
Generative AI might be applied to create natural language summaries of dashboards, or to allow stakeholders to ask questions about audience data via chat interface (e.g., “Which customer segment had the highest growth last quarter and why?” and get a coherent answer drawing from the data).
In essence, analytics tools are likely to become more like “analytics assistants,” accessible to non-specialists via AI.
Privacy and Data Ethics at the Center
The trajectory of privacy regulations will continue – with potential new laws and browser features further limiting individually identifiable tracking.
By late 2024/2025, Google Chrome is (projected) phasing out third-party cookies.
The industry is shifting to first-party data and consented ecosystems.
Techniques like federated analysis (where data doesn’t move, algorithms move to data), differential privacy (adding noise to data to protect individuals while preserving aggregate insights), and aggregated measurement APIs (like the Privacy Sandbox proposals) will likely standardize.
Audience analytics must adapt by focusing on trends and patterns at a cohort level rather than micro-level personal data.
This might slightly reduce the precision of targeting but could improve trust.
Companies that build transparent data practices and perhaps offer value back to users for their data (like personalization they genuinely appreciate, or loyalty rewards) will have richer data to analyze.
The future might also bring more consumer participation in data – e.g., personal data vaults where users control what data to share with companies, which analytics systems will need to interface with.
Cross-Device, Cross-Environment Integration
As new environments emerge (AR/VR, wearables, IoT), audience analytics will expand to those touchpoints.
The metaverse or spatial web analytics could become a thing – measuring how users navigate virtual 3D stores or how long they engage with a virtual concert.
Already, gaming engines have analytics for virtual world interactions; this could blend with traditional analytics if, say, virtual showrooms become part of retail.
The challenge of unifying data will grow as the number of devices per person grows (smart home devices, connected cars, etc.).
The goal remains a 360-degree view of the customer, but now 360-degree might be literal in AR contexts! Companies will invest in central data platforms and identity resolution techniques (possibly privacy-safe) to ensure they know that the John Doe who uses their mobile app is the same John Doe visiting their store and their VR experience.
Achieving this ethically and accurately will be a key competitive differentiator.
Real-Time Becomes Table Stakes
By 2025, many are doing near real-time analytics, but by 2030, real-time (or even predictive real-time) will be expected.
For instance, retail sites might use real-time analytics to alter pricing dynamically for each user context, or media platforms might adjust content almost instantaneously based on viewer sentiment (some experimental interactive storytelling is heading this way).
Event-driven architectures and streaming data technologies (like Kafka, Spark Streaming) will be commonplace under the hood of analytics systems, enabling continuous analysis rather than batch reports.
This could blur the line between analytics and action – the moment analytics detects something, automated triggers might execute (e.g., detect a user struggling on a website -> proactively pop up a help chat).
Emphasis on Value and Actionable Insights
The “helpful content” analogy applies to analytics too – it’s not enough to collect data, companies will judge success by the clarity of insight and the action taken from it.
The future will likely see tighter integration between analytics insights and execution platforms. Already we have marketing automation that uses analytics segments to trigger emails.
This might extend: analytics finds a cluster of customers at risk of churn and directly feeds a system that auto-generates a personalized retention offer for them, possibly even testing different offers and learning which works best.
Closed-loop analytics (where the insight immediately leads to an intervention and the results of that intervention feed back into analytics) will be a hallmark of advanced organizations.
Industry-Specific Advance
Different sectors might develop their own analytics standards and innovations.
For example, in healthcare, patient journey analytics including wearable data could revolutionize personalized medicine insights (with careful privacy handling).
In education, learning analytics might get very advanced in tracking student engagement and outcomes across digital platforms.
In government and smart cities, citizen data could be analyzed to improve services (e.g., traffic analytics leading to dynamic traffic light adjustments).
Each of these will rely on the same core advances (AI, integration, privacy) but applied in domain-specific ways.
Ultimately, the companies that will thrive are those who adopt a data-driven culture – not just having the tools, but making decision-making deeply intertwined with audience insights.
This includes upskilling staff to be comfortable with data, breaking down silos so that insights are shared broadly, and aligning KPIs across departments with measurable audience outcomes (customer satisfaction, engagement, conversion, etc., not vanity metrics).
In conclusion, the state of global audience analytics in 2025 is one of robust growth and rapid evolution.
We see clear trends of AI integration, real-time analysis, holistic cross-channel tracking, and personalization, all tempered by a growing emphasis on privacy and data ethics.
Across sectors – be it streaming media, social platforms, retail, events, or mobile apps – organizations are leveraging analytics to get closer to their audiences: understanding their needs and behaviors with unprecedented clarity and speed.
The market data confirms strong momentum, with the audience analytics industry expected to continue double-digit growth annually as businesses invest in tools and talent to harness data.
For any business or analyst looking at 2025 and beyond, the imperative is to stay user-centric: use analytics not for vanity or surveillance, but to genuinely enhance user experiences and provide value.
That aligns perfectly with what search engines like Google are pushing via algorithms favoring helpful content – the idea extends to analytics: insights should drive helpful outcomes for users, not just more pushy marketing. When done right, audience analytics is a win-win: users get more relevant, engaging experiences, and organizations achieve their goals more effectively.
This symbiosis is why audience analytics has moved from a niche function to center stage in corporate strategy.
As we progress, those who manage to navigate the technical, ethical, and analytical challenges will reap the rewards of deeper customer loyalty, more efficient operations, and the agility to innovate in a fast-changing market.
The narrative of 2025 is clear – data-informed understanding of audiences is no longer optional, but foundational to success in virtually every industry.